AI in Community Banking: Finding the Right Fit
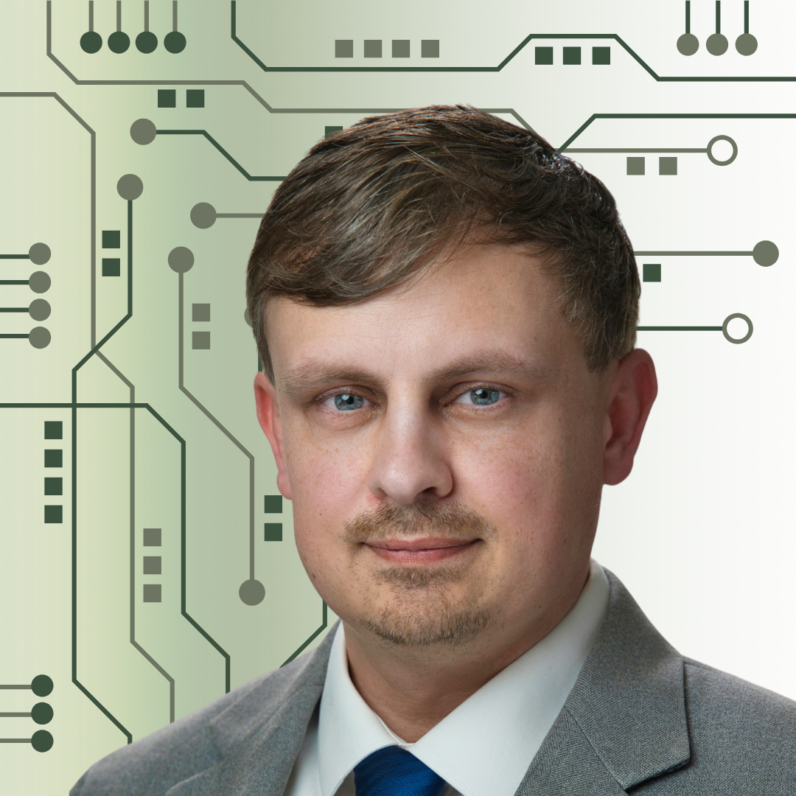
This column by Farmers & Merchants Bank CIO Tyler Morgan originally appeared in the October 2024 edition of Arkansas Money & Politics. Click here to see the magazine’s digital edition.
By Tyler Morgan
Chief Information Officer
Farmers & Merchants Bank
Across the banking sector, leaders are weighing AI’s immense potential against the risks it may pose to privacy and security. Like many of my fellow bank chief information officers and chief information security officers, I’ve seen an exponential increase in services promising the utopia that AI will bring. But it is not a panacea, and we must guard against trapping ourselves in a fool’s paradise.
Modern AI
AI might seem like a recent phenomenon, but its roots date to the mid-20th century. In fact, the term “artificial intelligence” was coined at Dartmouth in 1956. The late 20th and early 21st century saw advances in so-called narrow AI, which focuses on a specific subset of problems, versus the broader AI that has consumed the tech space in the past few years.
It is this more general and cognitively advanced AI that has sparked a mix of excitement, fear, obsession and paranoia. ChatGPT, Google Gemini, Claude and other generative AI services prompt such emotion due to their ability to inspire — and terrify — based on their humanlike reasoning, conversation and creative abilities. Moreover, concerns around AI are compounded by the ever-increasing number of data breaches and data mishandling incidents at firms across many industries.
Banks themselves are not AI newcomers; they used narrow AI for things like fraud detection, consumer loan decisioning and tailored chatbots many years before the release of ChatGPT. What has changed — almost overnight — is the introduction of generative AI models into banking platforms. Whether through third-party models like ChatGPT, or proprietary models, fintechs and banks are exploring myriad ways to leverage this exciting technology.
Finding A Good Fit That Mitigates Risk
When selecting an AI service, banks should select proprietary or paid models that provide data privacy and security controls. Whether a generative AI service is free or paid can directly affect the privacy and security commitments of the vendor offering the service.
For example, while Google Gemini is free, it is subject to Google Workspace enterprise data
privacy and security controls if purchased as part of a Google Workspace enterprise license. This means that data fed to the model is not aggregated for other purposes (such as model training) and is kept proprietary to the organization that licensed it. Likewise, upgraded plans with OpenAI’s ChatGPT and Anthropic’s Claude offer more control over the use of private data within those models.
When selecting a vendor that uses generative AI, banks should also confirm that the vendor has taken the same precautions to ensure that proprietary information isn’t leaked or absorbed by a model that lacks adequate security controls and later exposed in a breach.
Banks must also balance compliance concerns. If generative AI is used in a credit decisioning model, banks run the risk of implementing a model that may contain an underlying bias, and thereby negatively affect consumers. Such bias can be difficult to detect in a generative model because the model itself is more complex than traditional rules-driven algorithms. As a result, a bank may face steeper challenges in terms of demonstrating that its decisioning model is valid and doesn’t exhibit inherent bias.
When it comes to phasing in generative AI, banks should seek low-risk use cases that avoid
areas of heavy regulatory concern. This will allow banks to “learn as they go” and introduce
generative AI into more complex use cases methodically. Banks should also ensure that a bank employee is assigned to continuously test and monitor the AI’s behavior for quality assurance purposes.
There are several initial AI use cases that would allow banks to cut their teeth on the technology without taking too much risk:
● Using AI to review vendor contracts for specific terms.
● Using an AI chatbot to respond to employee searches for content in policies or about
bank product offerings.
● Using AI chatbots for limited customer service, such as pointing customers to self-
service resources on the bank website, locating bank phone numbers, responding to
questions about the bank’s open/close time, or supplying the bank’s routing number.
● Using AI in the back office to assist with solving problems around automation, such as
“assisting” a bank’s IT staff with scripting automations for certain processes.
While the AI frontier is fraught with security and privacy challenges, banks and their customers have much to gain if they can implement AI responsibly. Community banks, working carefully and deliberately, can help lead the way.
Tyler Morgan is the chief information officer of Farmers & Merchants Bank of Stuttgart.